A Business Leader’s Machine Learning Guidebook – Part 1
“Machine learning is here. It will make everyone’s lives easier and every company more productive. With the help of machine learning, each of us will have a digital twin to do all the boring work for us. And machine learning is so easy to implement and to deploy, thanks to all the tool vendors and open source libraries that offer us a huge array of ready-to-use machine learning algorithms. You do not even need a data scientist anymore: it is enough that you identify a business problem, and then anyone can develop and deploy a robust end-to -end machine learning solution within weeks, or even faster.”
This sounds great! But if machine learning is this easy, why hasn’t my company implemented it already?
Let’s start with a business problem. Say I would like to implement a machine learning solution that monitors the machinery in my factories and can inform me in advance if a machine is going to fail.
We skip forward in the story: now it’s nearly a year later, we have spent several hundreds of thousands of euros, and our machine learning solution is ready. I can now monitor the temperature, moisture, vibration, etc., and also record the sounds of the factory machinery. We are even transferring all the alarms and status data from the machinery to our data lake in the cloud. We actually already have terabytes of data and nice visualisations and reports from all the key data. And as you know: “data is gold.”
Really cool! So, can I now predict when my machines are about to fail?
Well, we first tried with our own staff, but predicting the failure of a machine has turned out to be more demanding than we expected. So, a couple of weeks ago we hired an external data scientist. She said it is not possible to make any predictions about a failure if we cannot model the situation where the failure occurs. She also said we would need some domain experts to explain what the data means. And she mentioned something about a computational problem that should first be defined.
The external data scientist also thinks that the current data collection process needs to be modified. In her opinion, it is lacking some essential information. She thinks that most of the data in our data lake might be useless.
Always beware of the hype
But wasn’t I told that machine learning was easy? And that we do not need data scientists anymore?
This story is of course fictitious, but it contains a big seed of truth. While machine learning, AI, and data science do offer new revenue and cost-saving opportunities, due to the massive hype around them, they also present a risk of failed investments.
One of the most important things to consider before moving forward with a machine learning project is the identification of a relevant business problem. This business problem needs to be able to be translated into a computational problem, or a machine learning problem. The business case of the problem should be also estimated before moving forward. Furthermore, it is a team effort where you need people with versatile backgrounds and knowledge.
So now that we’ve had a cautionary example and know what not to do – in the next part we shall move on to tips and best practices to succeed!
About the Author:
Jyrki Martti, Senior Advisor, is responsible for developing Sofigate’s data analytics concepts. Jyrki is interested in data science and machine learning. And especially how companies, municipalities and other organizations can develop their operations by the means of data analytics and machine learning.
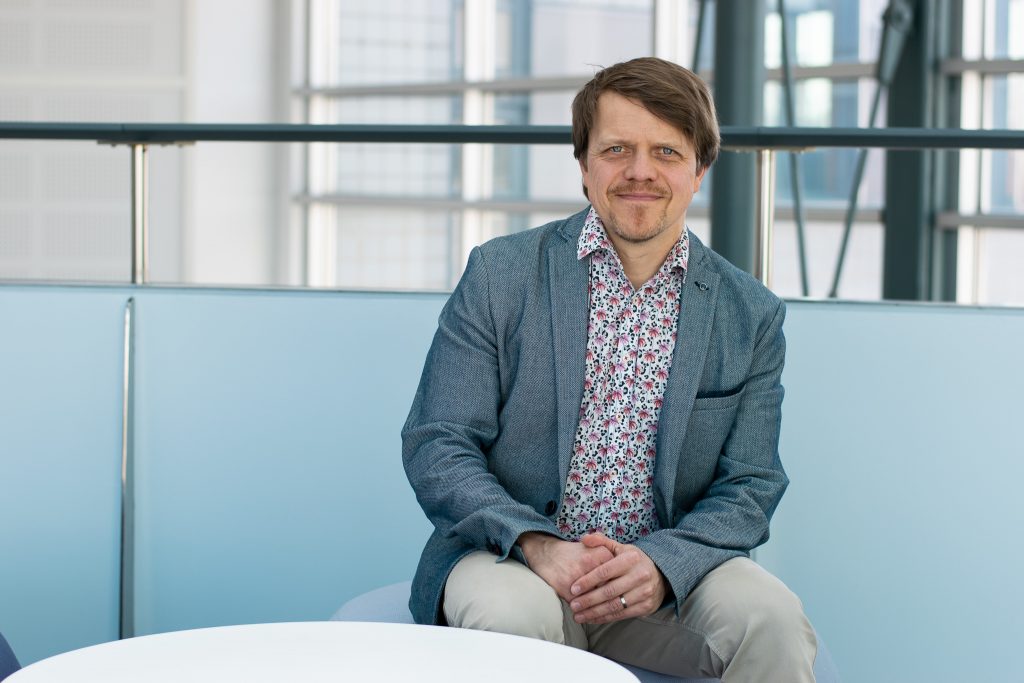
Jyrki Martti