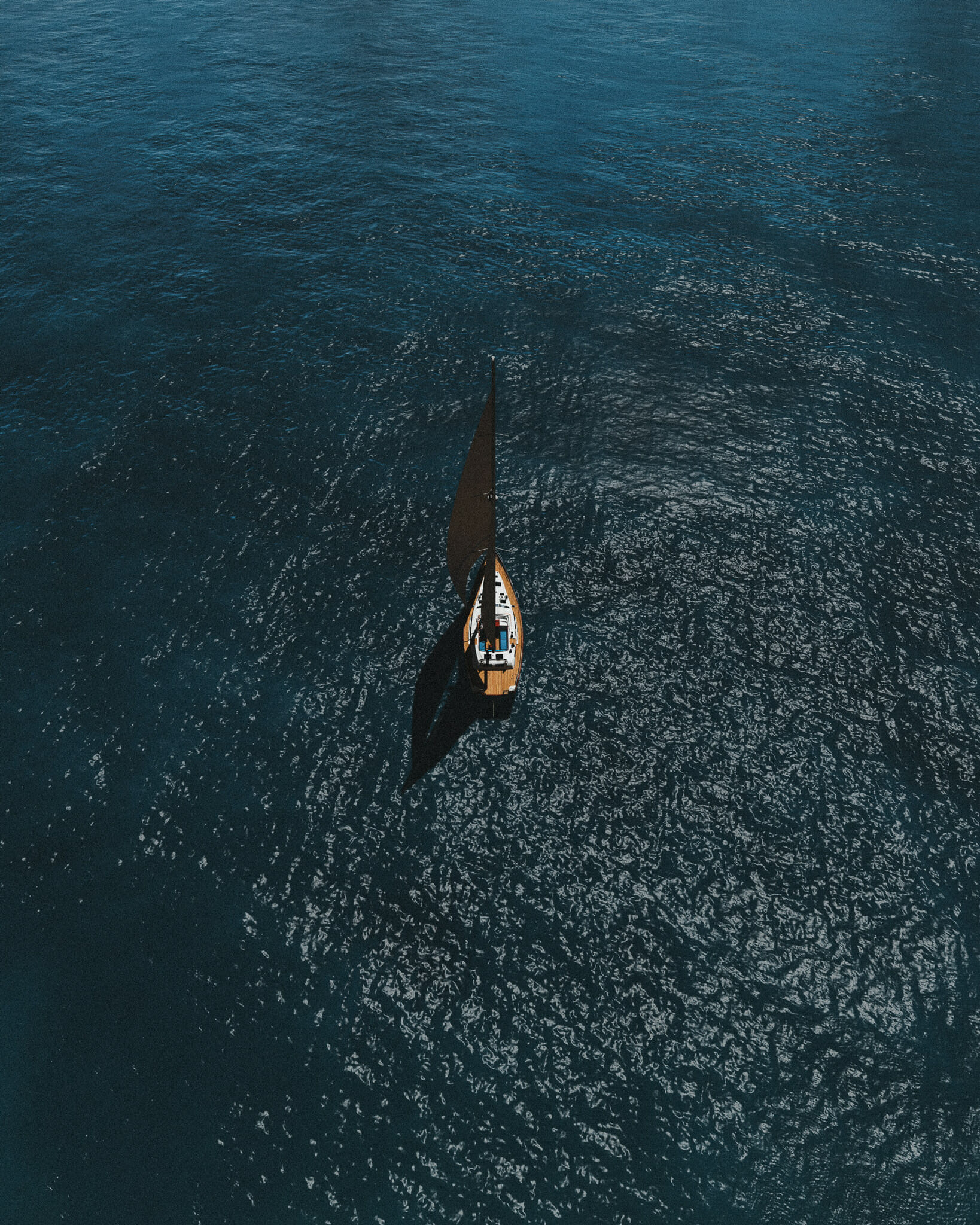
Insights
Want to learn something new?
You’ve arrived in the right place – here you can find our blogs, news & more
Blog
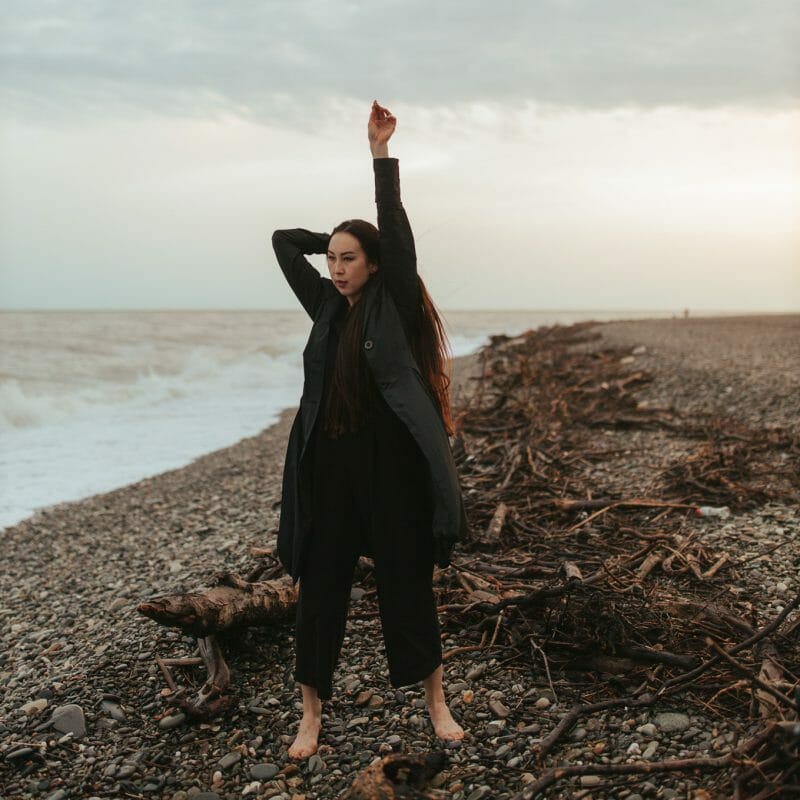
News
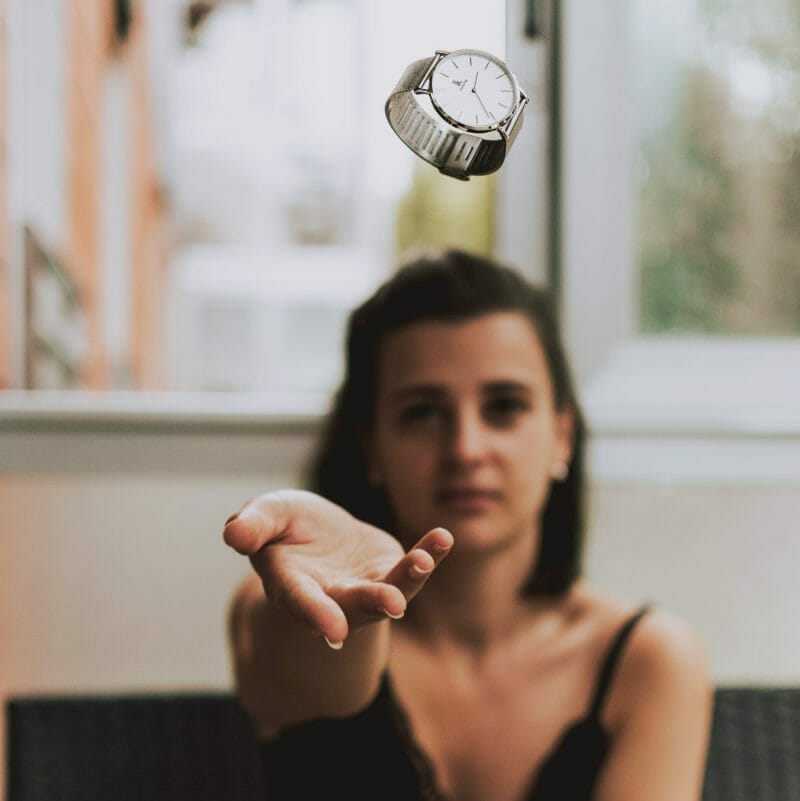
References
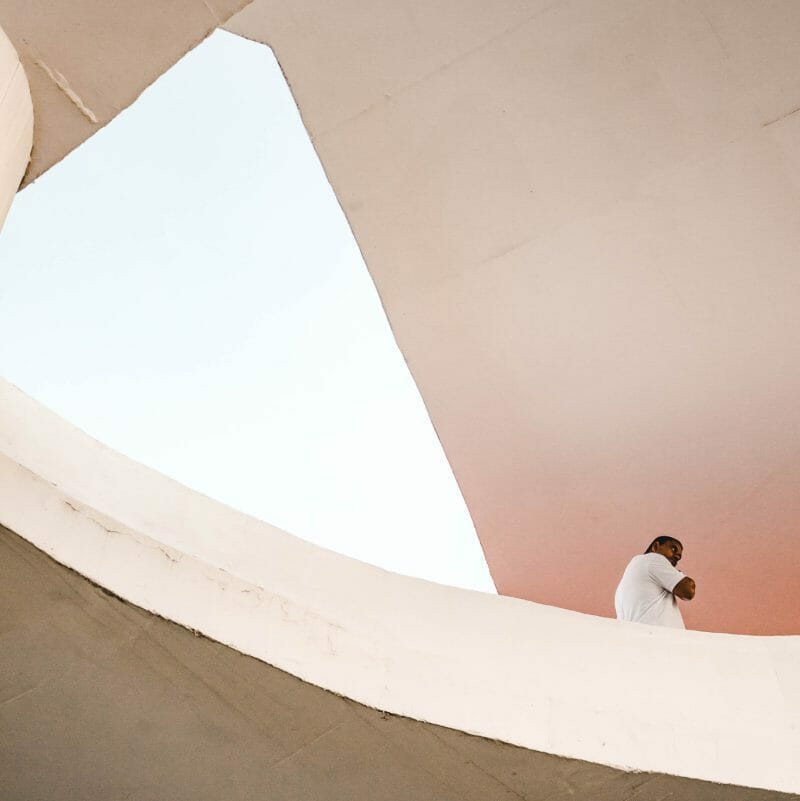
Not finding what you’re looking for?
We’ll be happy to help – send us a message
Want to learn something new?
You’ve arrived in the right place – here you can find our blogs, news & more
We’ll be happy to help – send us a message